Recently, DUT-RU ISE research team got their results in the field of multi-modality image fusion accepted by the International Journal of Computer Vision (IJCV), a top journal in the fields of artificial intelligence and computer vision. The paper titled “CoCoNet: Coupled Contrastive Learning Network with Multi-level Feature Ensemble for Multi-modality Image Fusion” was completed by Dr. Liu Jinyuan, postgraduates Lin Runjia and Wu Guanyao, Prof. Liu Risheng, Prof. Luo Zhongxuan and Prof. Fan Xin (corresponding author). It is the latest research achievement made by the Institute of Geometric Computing and Intelligent Media Technology.
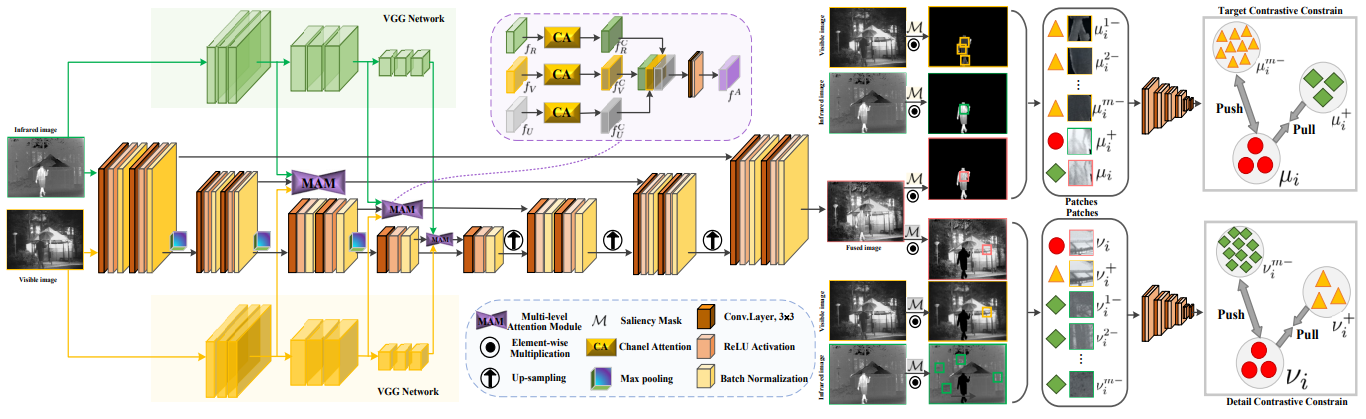
Multi-modality image fusion obtains fusion images with much richer information and higher quality by integrating complementary information obtained from different sensors, which is of great significance for enhancing vision and improving image semantic analysis and application. The team found that existing deep learning multi-modality image fusion algorithms mainly preserve complementary features by constructing various loss functions, but usually ignore the intrinsic connections between different sensing modalities, resulting in redundant or invalid information in the fusion results. To solve this problem, this paper innovatively proposed a coupling contrast constraint to optimize the loss function, so that the foreground target/background details of the fusion image and the corresponding infrared/visible light source image are closely connected in the feature representation space while being far away from the features of another modality, effectively avoiding distortion in the fusion process. Additionally, the paper introduced a multi-level attention module to learn rich hierarchical feature representations and comprehensively convey semantic information features during the fusion process, successfully promoting multiple image fusion tasks and subsequent semantic perception tasks. Relevant results have been open-sourced at https://github.com/runjia0124/CoCoNet.
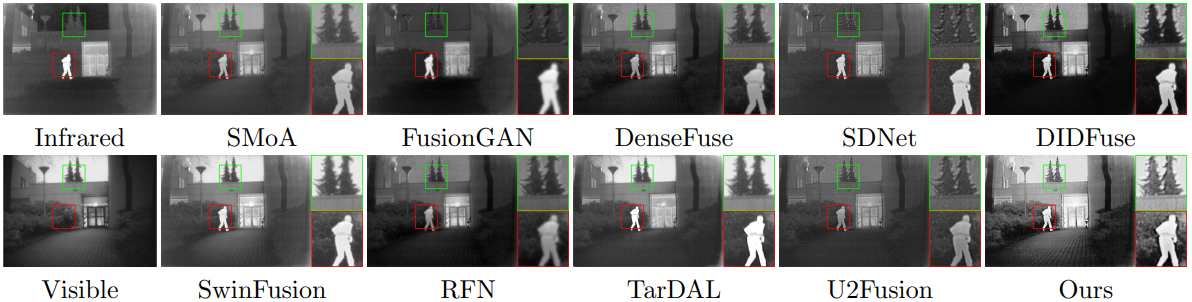
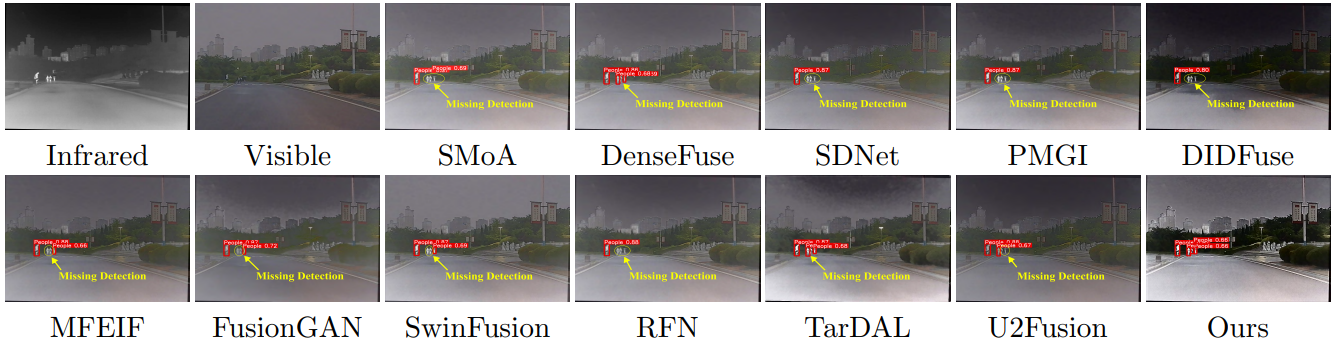